Novel view synthesis(NVS)
最近刚接触到这个话题,想开一篇新博客来记录一下自己学习的过程。
首先我是看了两篇review了解了这个topic的主要任务:
- advancements in radiance field techniques for volumetric video generation: a technical overview
- From Capture to Display: A Survey on Volumetric Video
另外同步阅读了huggingface的tutorial:https://huggingface.co/learn/computer-vision-course/unit8/3d-vision/nvs。这篇博客将NVS描述为这样一个任务:
generate views from new camera angles that are plausibly consistent with a set of images.
我们在对一个场景进行3D还原时,首先的输入是一系列相机在不同的视角拍摄的静态图片,通过这些图片我们对该场景下的人物以及物体进行3D建模,但相机个数是有限的,如何推算出某个没有相机的角度上的view,这就是NVS这个任务要做的事情。
很多方法在这个topic上提出来,大致可以分成两类:1)generate an intermediate three-dimensional representation, which is rendered from a new viewing direction. 比如PixelNeFRF 2)direclty generated new views without an intermediate 3D representaion, 比如Zero123
NeRF
NeRF: Representing Scenes as Neural Radiance Fields for View Synthesis
2020年出的一篇文章,下面这句话就是它这个算法的精华:
Our algorithm represents a scene using a fully-connected (nonconvolutional) deep network, whose input is a single continuous 5D coordinate (spatial location (x, y, z) and viewing direction (θ, φ)) and whose output is the volume density and view-dependent emitted radiance at that spatial location
LLFF 数据集
在查看NeRF的github code时,发现作者使用了两个数据集,其中一个就是LLFF,本着学习的原则,先把LLFF数据集搞清楚。
LLFF全称为Local light Field Fusion,也是提出了一个NVS的算法。LLFF的主旨思想是:
present a simple and reliable method for view synthesis from a set of input images captured by a handheld camera in an irregular grid pattern.
简单说就是:该方法可以从一系列手持拍摄的静态图片生成一个scene,这个scene可以理解为一个3D的场景,可以用VR眼镜看的那种。
该LLFF repo提供了非常详细的安装教程,令我比较感兴趣的是,它可以基于自己拍摄的一些静态图片生成一个scene。先来看看它的这份代码。
我们的输入是从一系列的images开始的,首先第一步
- recover cammera poses
这一步采用COLMAP 实现了一个 struture from emotion + Multi-View Stereo(MVS)的完整pipeline。这一步的输入是一系列的静态图像,输出的是这个场景下的 6-DoF camera poses和 near/far depth bounds。
Structure-from-Motion (SfM) is the process of reconstructing 3D structure from its projections into a series of images. The input is a set of overlapping images of the same object, taken from different viewpoints. The output is a 3-D reconstruction of the object, and the reconstructed intrinsic and extrinsic camera parameters of all images.

COLMAP使用的方法依赖于Structure-from-Motion Revisited这篇文章,它将SfM分成三个步骤:
- feature detection and extraction
这一步好理解,特征抽取,利用一个apprearance descriptor f
- feature matching and geometric verification
feature matching利用前一步的features找出这一系列图片中的same scene part。原文是这样写的:
The na ̈ıve approach tests every image pair for scene overlap; it searches for feature correspondences by finding the most similar feature in image I(a) for every feature in image I(b), using a similarity metric comparing the appearance fj of the features
简单理解就是根据上一步抽取的features去一一比对每一对image pair,寻找出每一对image pair中的相似feature,从而找出same scene part。
geometric verification 我觉得有点稍微难理解。上一步只是确认了每一张图片中在apperance上相似的scene part,但有可能不是指代的这个场景下的同一个object(Point), 所以就需要去verify上一步的match是否准确,怎么verify呢?通过projective geometry去预估transformation
Since matching is based solely on appearance, it is not guaranteed that corresponding features actually map to the same scene point
- structure and motion reconstruction
Multi-View Stereo(MVS) takes the output of SfM to compute depth and/or normal infomation for every pixel in an image.Fusion of the depth and normal maps of multiple images in 3D then produces a dense point cloud of the scene.
实现脚本为imgs2poses.py
,
看该源代码就是基于COLMAP来做的。试着运行该脚本,测试数据用repo内的download_data.sh
下载的数据。运行完后可以看到如下输出:
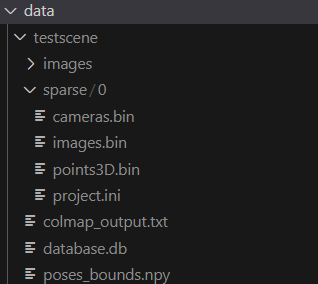
其中images是source images,testscene下除了images这个文件夹,其他都是COLMAP生成的。具体含义参考COLMAP的document
logs文件内容:
1 | Need to run COLMAP |
仔细分析一下imgs2poses.py
,一共用COLMAP执行了三条terminal命令:
1 | # extract features |
对照colmap cli的guidebook,
作者使用了前三个命令,dense部分没有继续生成。想要知道它output出来的这些.bin文件含义,需要搞明白database.db
内有什么东西,它是feature
extraction的产物。db文件可以用vscode的插件打开,它包含7个table:
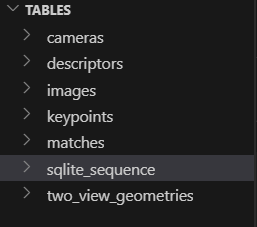
keypoints表格中,我下图截图的data部分里才是所有feature的信息,内有每一个feature所在的X,Y坐标。
COLMAP uses the convention that the upper left image corner has coordinate (0, 0) and the center of the upper left most pixel has coordinate (0.5, 0.5)
COLMAP在表示图像坐标时,采用了一种特定的坐标系统,其中图像的左上角被定义为坐标原点 (0, 0)。而“the center of the upper left most pixel has coordinate (0.5, 0.5)” 指的是图像中最左上角的像素的中心位置被赋予了坐标 (0.5, 0.5)。
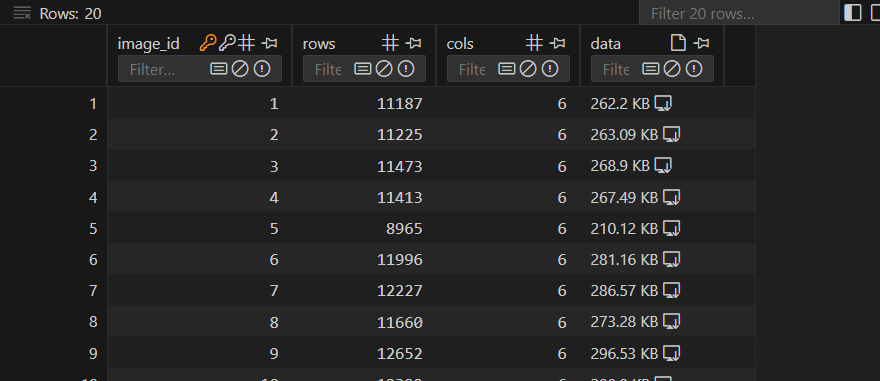
在这两张表格中,rows表示的数值是number of detected features per image, 如果rows=0, 那么这个image没有feature
在运行命令colmap exhaustive_matcher --database_path ./data/testscene/database.db
后,db文件内的matchs这张表会出现值(之前没有),每一行会表示一张图片和另外一张图片的匹配结果,rows的值表示match上特征点的个数。